Imagine you’re a marketer at a growing e-commerce company, and your goal is to enhance customer engagement and increase sales. However, your diverse customer base makes it challenging to deliver personalized marketing messages effectively. This situation calls for a proper segmentation strategy. In this blog post, we’ll explore three effective segmentation techniques: pre-defined business rules, supervised segmentation, and unsupervised segmentation, providing actionable insights to transform your marketing efforts.
The situation you are in is that your marketing campaigns aren’t getting the expected results. Your email open rates are low, and the click-through rates are disappointing. You realize that sending generic messages to your entire customer base is not working, you need a more targeted approach. The task here is to segment your customer base into distinct groups that share common characteristics. This targeted approach allows you to tailor your marketing to each group’s unique needs and preferences, improving engagement and conversion rates. Sounds easy right?
In today’s world of advanced data analysis, segmentation extends far beyond the traditional 2×2 consultancy matrix. Organizations often mistakenly presume that segmentation only involves high versus low revenue, profits, market share, etc. Unfortunately, this approach does not solve our problems. While these matrices can be useful in straightforward situations, they fall short in capturing the nuances of real-life data. Our approach to segmentation typically involves multiple dimensions – often more than four – to identify complex, tangible groups. With various methods we simplify the inherent complexity of real-world data, making it more manageable and more importantly actionable. So what are our actions? We typically tackle these problems with three methods namely: Pre-defined Business Rules, Supervised Segmentation, Unsupervised Segmentation.
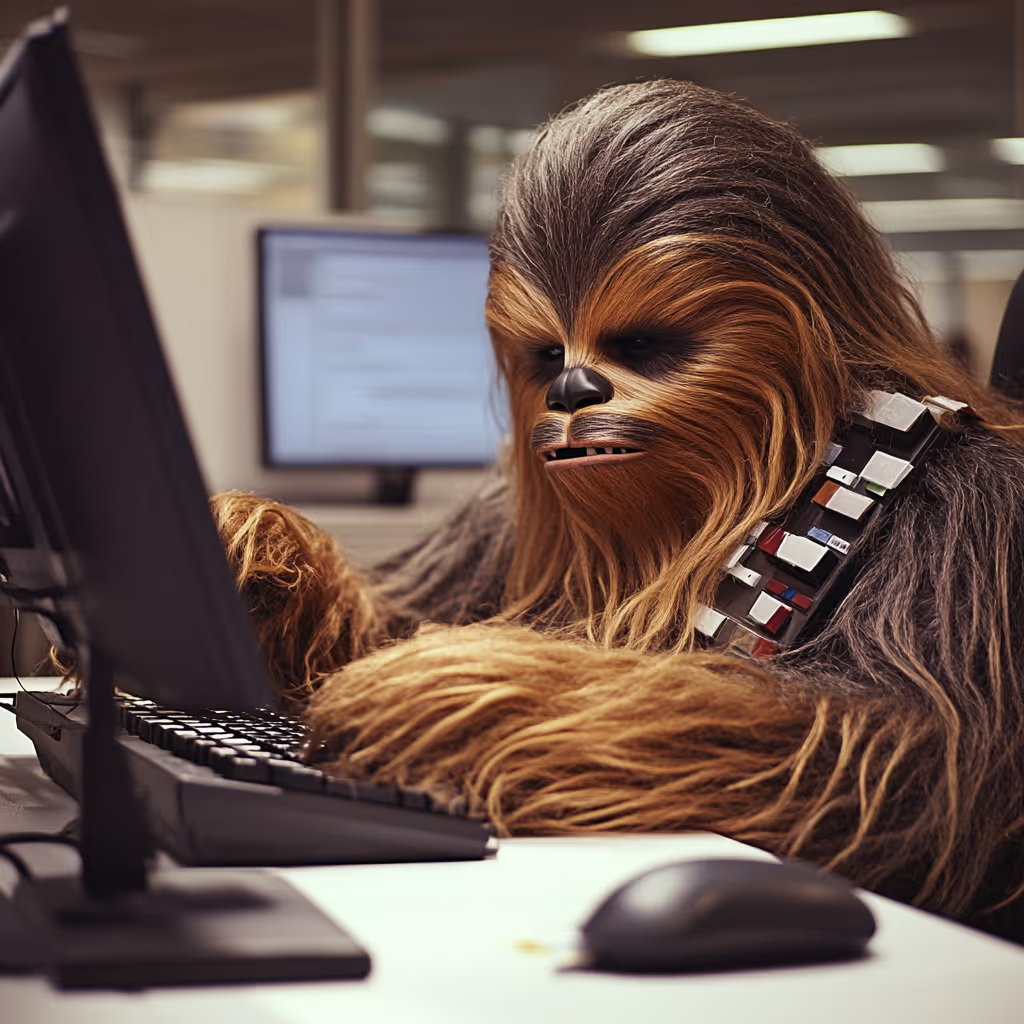
Pre-defined Business Rules
Pre-defined business rules use static criteria based on existing business knowledge to segment data. One commonly employed method is the traditional 2×2 consultancy matrix, where customers are categorized by factors such as profitability and sales volume. This results in segments like high profit/high sales, high profit/low sales, low profit/high sales, and low profit/low sales, each receiving tailored marketing strategies. While this approach is straightforward and easy to implement, it often fails to capture the complexity and nuances of real-world data. It tends to oversimplify, ignoring interdependencies with other factors, such as the impact of weather on sales and profitability, and lacks depth. Another frequent example is geographical segmentation by regions (e.g., South, West, East, North), allowing organizations to address regional preferences and market conditions. This model provides clear and manageable segments for delivering targeted marketing messages. However, its simplicity can lead to the oversight of other influencing factors.
Now, let’s talk about a more advanced rule-based approach that adds sophistication to our segmentation efforts: the RFM (Recency, Frequency, Monetary) model. This method dives deeper by looking at three critical factors: the number of days since a customer’s last purchase (Recency), how often they make purchases (Frequency), and how much money they’ve spent (Monetary). By combining these three metrics, organizations can identify various segments, such as loyal customers, champions, or customers at risk of leaving. This method is straightforward and highly effective for quick operational decisions, especially when advanced segmentation models are not in place. In our next blog, we will discuss the RFM model in more detail, exploring how you can leverage pre-defined business rules to direct your marketing efforts.
Unlike algorithms or machine learning models, pre-defined business rules are crafted by business stakeholders, drawing on their knowledge, experience, and industry standards. This approach is not a machine learning method; instead, it relies on predetermined criteria to segment customers. The simplicity behind these models makes them easy to implement and understand, but they may not always provide the best results.

Supervised Segmentation
Supervised segmentation uses labeled data to train models that recognize patterns, helping you predict future actions and classify customers into categories such as “loyal” or “at-risk of losing.” By leveraging historical customer data, this approach ensures accurate segmentation of new data.
Imagine training a new employee to sort emails into “Spam” and “Not Spam.” You start by showing them examples of both, explaining why certain emails are classified as spam due to keywords like “free money” or suspicious senders. Over time, the employee learns these patterns and can sort new emails independently. Similarly, supervised segmentation relies on trained models that recognize patterns from labeled data.
The purpose of supervised segmentation is to use existing information to accurately categorize or segment new data. This approach is particularly useful when you have labeled examples and want to classify new data similarly. When it comes to filtering spam emails, various methods can be employed. Some are straightforward, involving basic probabilistic or regression methods, while others are more advanced, requiring significant computational resources and leveraging complex decision-making processes. For even more sophisticated filtering, iterative improvement techniques and advanced models capable of capturing intricate patterns found in email data can be used.

Unsupervised Segmentation
Unsupervised segmentation uncovers hidden patterns in data without the need for labeled examples. By clustering customers based on similarities, such as purchase behavior or browsing history, you can identify natural groupings. This method is particularly useful for exploring the “hidden” structure of data when there are no predefined categories.
Imagine giving an employee a stack of emails and asking them to sort them into groups without any instructions. The employee might notice some emails contain offers for free bitcoins, others come from familiar senders, and some discuss various services. Based on these observations, they’ll group the emails accordingly, creating clusters that they infer to be spam and non-spam.
The goal of unsupervised segmentation is to uncover hidden patterns or groupings in data without any prior labeling. This approach is beneficial when you don’t have predefined categories and want to explore the natural structure of your data. Various unsupervised learning methods can be employed to discover these underlying patterns. Some techniques are simple, grouping similar data points by iteratively adjusting cluster centers. Others are more complex, building nested clusters in a tree-like structure and merging similar items step-by-step.

So what’s in it for me?
By employing these segmentation techniques, you can develop more targeted marketing strategies. For a leading retailer, these methods led to more engagement and almost doubled the return on ad spend within the first three months. Email campaigns now deliver personalized content, resulting in higher open and click-through rates, increased sales, and customer satisfaction (measured by net promotor scores, NPS) is on the rise because the messages resonate more with each customer segment.
Looking Ahead: What’s Next in This Series
You’ve just read Part 0 and Part 1 of our series on segmentation in marketing, where we explored the what, why, and how of customer segmentation. In the upcoming parts, we will delve deeper into practical applications, tools, and case studies to transform your business insights into actionable strategies.
What to Expect in Part 2 (Coming Soon)
In the second part, we will focus on applying segmentation in an online retail context. Here’s what you can look forward to:
- Data Collection and Management: Some basic techniques and best practices for gathering and organizing customer data specific to online retail.
- Rule-Based Segmentation: Applying a simple RFM model to segment customers based on purchasing behavior.
- Practical Applications:Step-by-step guides and practical examples for implementing RFM strategies in an online retail setting.
This part will provide actionable insights that online retailers, big or small, can use to enhance their marketing efforts and customer engagement.