Artificial Intelligence (AI) has become a buzzword in recent years, mentioned by many but rarely understood. What exactly is AI? and how does it work? In this blog, we’ll explore the fascinating world of AI, shedding light on its fundamental principles and mechanisms.
In our previous blog, we jumped into the intriguing concept of machine learning. We uncovered four major branches: supervised, unsupervised, semi-supervised, and reinforcement learning. Each possesses the incredible ability to learn without human intervention or explicit programming.
In this blog, we get a little more technical. We’ll discover some key tasks these intelligent systems can perform to help us understand the world around us in a more structured way. The tasks we are referring to are called classification, regression, clustering, and dimension reduction.
Before we dive into the specifics, let’s revisit the term labelled data and supervised learning. Imagine teaching a child to recognize animals by showing them pictures and naming each one. This process is quite similar to how machine learning, uses labelled data. In the world of machine learning and AI, labelled data acts as a guide, where every piece of information (like a photo of an animal) comes with a correct answer or label (such as “Dog” or “Capybara”). In a professional context, predicting if a customer will churn or if a patient has a specific disease, are good examples. For now, remember that when we have labelled data, we can use supervised learning. Otherwise, we need to employ different methods and techniques.
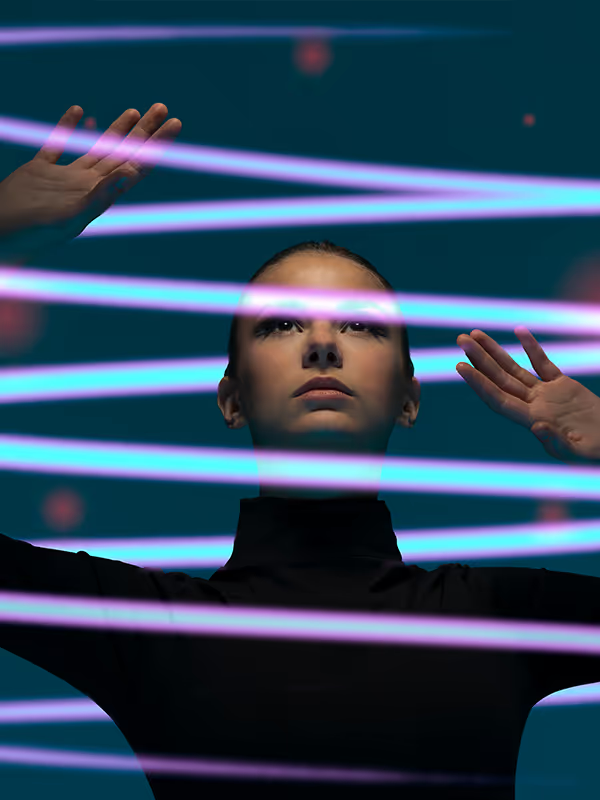
Understanding Classification | supervised machine learning
Classification is like sorting your cloths after doing the laundry. Once the washing machine has finished and everything is dried up, you sort your socks, underwear, shirts, and trousers into different piles within your Ikea Pax closet. Classification algorithms help us categorize data. It’s all about putting things into predefined groups, based on the information provided.
In the laundry example, we can distinguish between socks and shirts easily because you probably cannot pull a sock over your head. In this case, we predict or categorize a “sock” and place it into the right pile. The power of classification lies in its ability to learn categorical outcomes from examples where input data is paired with correct labels, predicting categorical values such as email spam detection (spam/not spam) or customer profiles (A, B, C, D). This makes it a valuable tool for testing potential business strategies.
The Role of Regression | supervised machine learning
Imagine a cafe that sells more hot chocolate in winter and iced tea in summer. By looking at sales data from previous years, alongside weather patterns, holiday seasons, and customer preferences, the owner can make educated guesses about how much of each item she/he will sell in the coming months. Regression analysis operates on a similar principle where it allows organisations to predict or anticipate future conditions by analysing historical data and considering variables such as weather conditions, market trends, and customer profiles. It is about drawing a map to the future, using the signs and signals from the past and present. Regression is also a supervised machine learning task. Like classification, regression tasks rely on labelled training data but focus on predicting numerical values such as prices, demand, or the number of customers visiting a store on a specific day.
The power of Clustering | unsupervised machine learning
Clustering is an unsupervised machine learning task that groups data points based on their similarity. Unlike classification or regression, it operates without labeled data and discovers patterns or structures within the data. The beauty of clustering lies in its ability to identify natural groups in the data without prior knowledge. At Chapter Data we typically use these techniques for exploratory purposes, such as identifying similarities in purchasing behavior to get a better understanding of customer profiles, groups or product portfolio.
The Importance of Dimension Reduction | unsupervised machine learning
Machine learning thrives on data, but not all data is equally valuable. Bringing in more training data does not necessarily yield better results. In fact, an abundance of information often leads to increased computation cost, complexity, overfitting, and difficulties in visualization or reporting. This is where dimension reduction comes in. Dimension reduction is an unsupervised machine learning task that reduces the number of features or variables in your dataset while preserving its essential information. By simplifying data or models without sacrificing critical patterns or relationships, dimension reduction helps avoid overfitting, enables faster computation, and makes data visualization or reporting easier.
Conclusion
We went a little deeper into actual tasks performed by intelligent sytems. Classification and regression are supervised learning tasks. They enable the categorization of data into predefined groups and the prediction of numerical outcomes using labelled training data. These processes mirror everyday decision-making, from sorting laundry to predicting sales, leveraging historical trends and other variables.
On the other hand, unsupervised learning tasks like clustering and dimension reduction leverage unlabelled data to find logical patterns. With clustering we search in the data for certain “similarity” patterns without prior labels or knowledge. We use dimension reduction to reduce the number of features or variables in your dataset while preserving its essential information (simplifying it).
As we continue to demystify AI and machine learning, we open the door to a world of possibilities driven by data and analytics. By challenging the status quo and exploring new possibilities, we can look forward to an exciting future powered by these technologies.